Overview
CloningDCB is a dataset of synthetic driving sequences generated using the CARLA simulator. The driving tasks are performed by 40 individuals on both dynamic and static driving platforms. Driving is not random but based on orchestrated scenarios.
Each sequence includes RGB images accompanied by standard ground truth data (depth, optical flow, semantic and instance segmentation), ego-vehicle information, and, most notably, eye-tracking recordings.
CloningDCB may be used for research and commercial purposes. It is released publicly under the Creative Commons Attribution-Commercial-ShareAlike 4.0 license. For detailed information, please check our terms of use.
CloningDCB features more than 15 hours of curated driving scenarios and free driving performed by human drivers in a realistic cockpit with CARLA simulator.
5 weather variations for every driving scenario are also provided.
Ground-Truth
CloningDCB brings per-pixel ground-truth semantic segmentation, scene depth, instance panoptic segmentation, optical flow and real eye tracking. Check some of our examples:
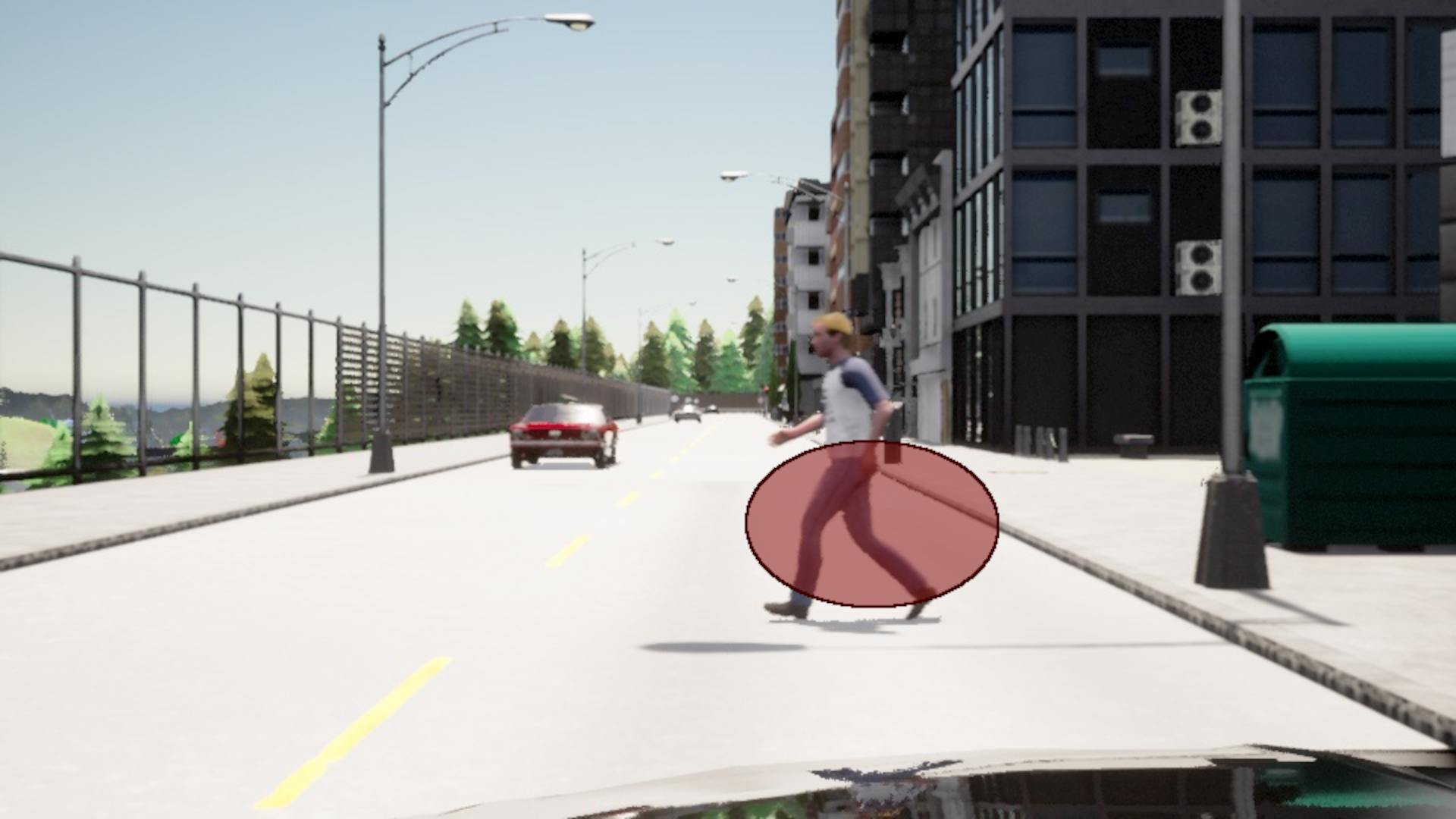